The Llama 3.2 launch brings a major stride in AI technology, especially in its Vision functions. This model is offered in two versions, one is of 11 billion parameters and the other with 90 billion parameters, with a view to improving both the text and the image processing. In this blog, we will look into the Llama 3.2 Vision features, its performance in different tasks, and the implications for AI apps.
Overview of Llama 3.2 Vision
Joining the Llama Vision with logic perception allows computers to be able to figure out the visual images and text outputs. With two different features, now developers are able to use applications to explain images and generate relative texts that users can understand easily. The titled Concept Best Together platform is more the common-used not-train mechanism. One way to solve the problem may be to switch the platform from the 90 billion parameter version to the multiple GPU environment. The 3.2 Vision of the Llama Llama Vision is a very smart and creative way to help computers to understand visuals based on logic.
Model Specifications
The Llama 3.2 Vision models are available in two configurations:
- 11 Billion Parameters: Suitable for lightweight applications and general use.
- 90 Billion Parameters: A more muscular design for complex tasks that belong to a high-grade accuracy category
Two models, one being optimized for speed and efficiency, are the great examples of AI that are real-time applications that can be used.
Testing Llama 3.2 Vision
Tests were run by Llama 3.2 Vision to clearly understand the vision and thereby the quality was also assessed. During the tests, the model’s skills were measured by its processing of exteroceptive input.
Initial Image Description
The initial test was a scenario in which the AI was asked to explain a simple image of a llama walking in a green field. It quickly and effectively identified the llama’s traits and the scenery. It shows you the model’s strong point of recognizing pictures like this.
Identifying Public Figures
Immediately hereafter, the model was tested on its capacity to identify a popular public figure, Bill Gates. The results were not good, as the model was unable to detect the person and thus claimed that no assistance in the identification procedure of people in images can be provided due to legal constraints. This fault can easily damage our reputation. It points to the flaw etiquette of the machine.
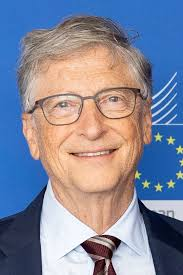
Captcha and Code Generation
It once more said it could not be of help with this request. Also, it passed on when requested to provide HTML coding for an ice cream selector app. This hyper-censorship appears to restrict the model from performing tasks that could be perceived as inappropriate.
Understanding Humor through Memes
Llama 3.2 Vision analyzed a meme that describes the differences between startup and corporate work cultures. In a more successful test, Llama 3.2 Vision was asked to explain a meme contrasting startup and corporate work cultures. The model provided a coherent analysis, indicating that it can understand and interpret humor in visual formats.
Advanced Image Processing Tasks
Going past the basic recognition, the model was probed with complex images, e.g., screenshots of data tables and QR codes. It worked well when an image of a table was transformed into CSV format. In particular, the model correctly detected the structure and contents of the data.
Storage Information Analysis
For this other task some colleagues made a recollection of an iPhone storage screenshot. The model succeeded in recovering the entire storage and free disk space, but the calculation of the available storage based on the full capacity of the data was incorrect. This proves that a procedure can prompt the model to manage individual questions. However, it may lose in the numerical accuracy dimension.
Challenges with Complex Queries
In one of the more difficult tests, the question the machine had to answer was to find Waldo in an intricate picture. Waldo was pointed to by the model to a place incorrectly. This failure stands out the difficulties Llama 3.2 Vision is undergoing while working with the most complex images such as the ones that require deep analysis.
Conclusion: The Future of Llama 3.2 Vision
On the one hand, Llama 3.2 Vision is an example of image recognition that is very well-made and is simple. On the other hand, the wrong way in which it censors certain queries makes it less accurate in really difficult cases. So what if it is telling raw, unfiltered truth ? – just a point to ponder, for sure. You must be wondering why Llama 3.2 Vision is considered silent. The censorship in the frequency of queries one makes lessen its functioning in doing complex tasks. Generally, the toolkit
The upcoming developments in AI technology bring the balance between security and optimal functionality as a major issue. The current drawbacks in Llama 3.2 Vision might be the driving force to come up with more changes and updates to enhance the product’s performance and customer experience.
For developers who want to take advantage of Llama 3.2 Vision, it is important to keep in touch with what it can and cannot do. By understanding it, developers will be able to make applications that are as good as the model can be, while avoiding its limitations.
Stay tuned for AI Advancements and the way they can change several industries. More updates are coming.