NVIDIA self-certified openAI Llama 3.1, a neural networking program with 70 billion parameters, which touched off a revolution that got AI developers and customers to rethink creativity and exhaust all possible mediums for their projects before risking the strong competitive ability the non-open-source route may have. Produced by CUDA, it’s a complex production setup that involves thousands of electronic parts some of which; in contradiction to what opponents might say; tend to be less powerful while the others are plasmoids that deliver most of the program’s calculations that make it function.
Introduction to Llama 3.1 Neaton
The Llama 3.1 mockup for Neaton 70B as a leading participant in the LM Arena AI Hard Benchmark shows a regimen that gets to the top of the leaderboard in the AI arena. The performance of this model not only excites but also indicates a quantum leap in the world of open-source AI technology. For example, NVIDIA adopted the base Llama 3.1 model and brought the model to the next level through quite advanced techniques such as reinforcement learning.
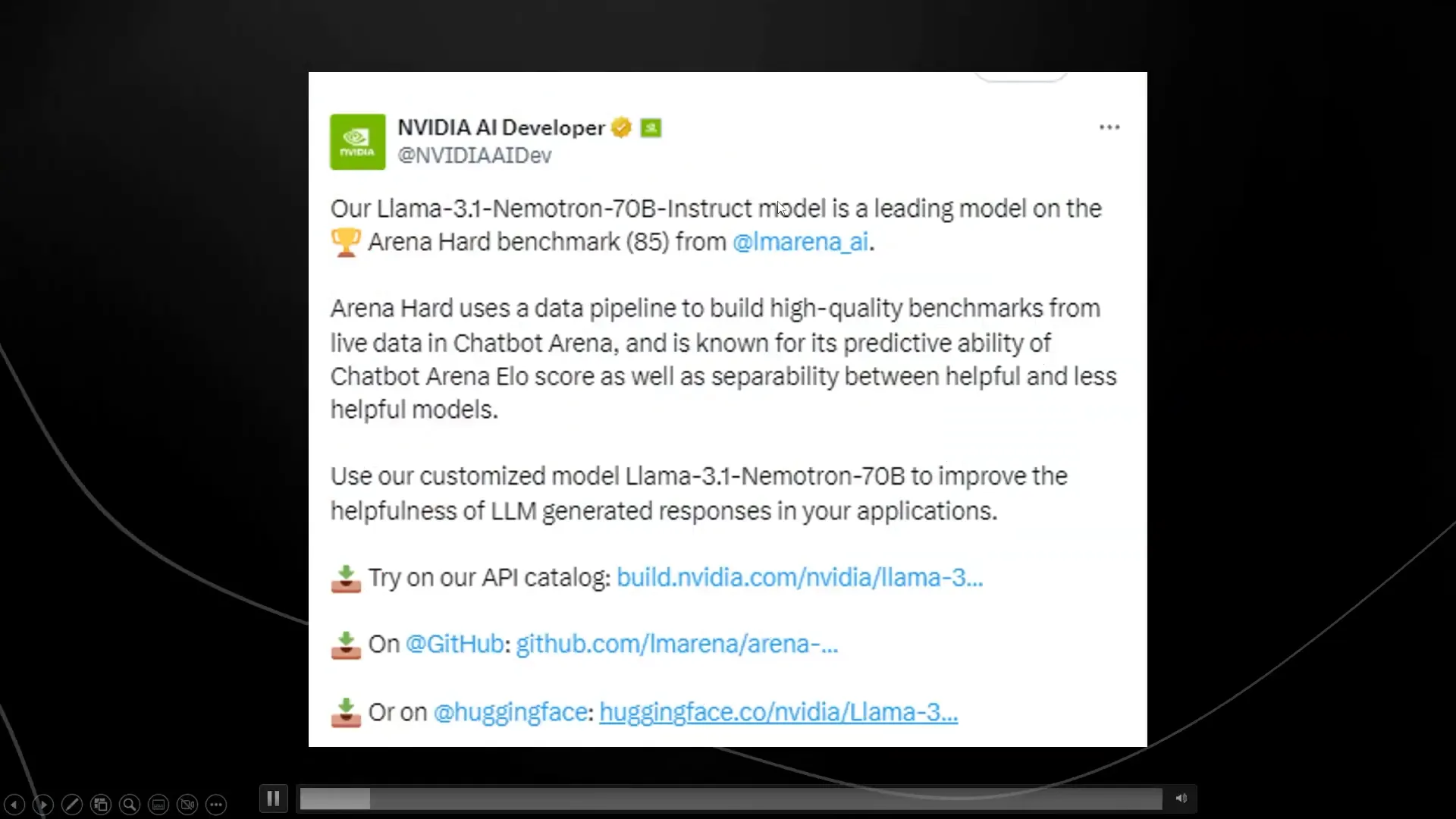
Benchmark Performance
The Llama 3.1 Neaton model stands apart with its benchmark performance, which is a real advantage. It scored 85% on the MT bench, which is not only the highest score ever but also greater than the equally new GPT-4 from OpenAI. One thing to point out is that this score is also better than that of Llama 3.1 45B instruct model whose size is much more.
The fundamental point how the training model is done is the main factor here. NVIDIA’s way of training models has been successful in enabling them to accomplish tasks bigger than closed-source designs, and occasionally best the latter. This achievement conveys the message that models are being trained and how they do, quite fundamentally, have influences on their effectiveness.
Innovations in Reward Modeling
Llama 3.1 Neaton is the breakthrough innovation with a difference that mainly lies in its core of more sophisticated reward modeling. The Bradley-Terry style and regression style were the two main types of modeling that were experimented with by NVIDIA’s scientists. Both methods are meant to steer the AI models to offer better and more useful responses by giving them reward scores that are based on their ability to fulfill the instructions.
The Bradley-Terry model is a way that is used to compare prompts in order to know which the best one, as a result, a the regression model is able to forecast a numerical score that tells the operator about blood iron levels such as helplessness and correctness for proteins for the winds. But a high level problem in connection with models, where they are often trained on various types of data, represents which ruins direct comparisons this way.
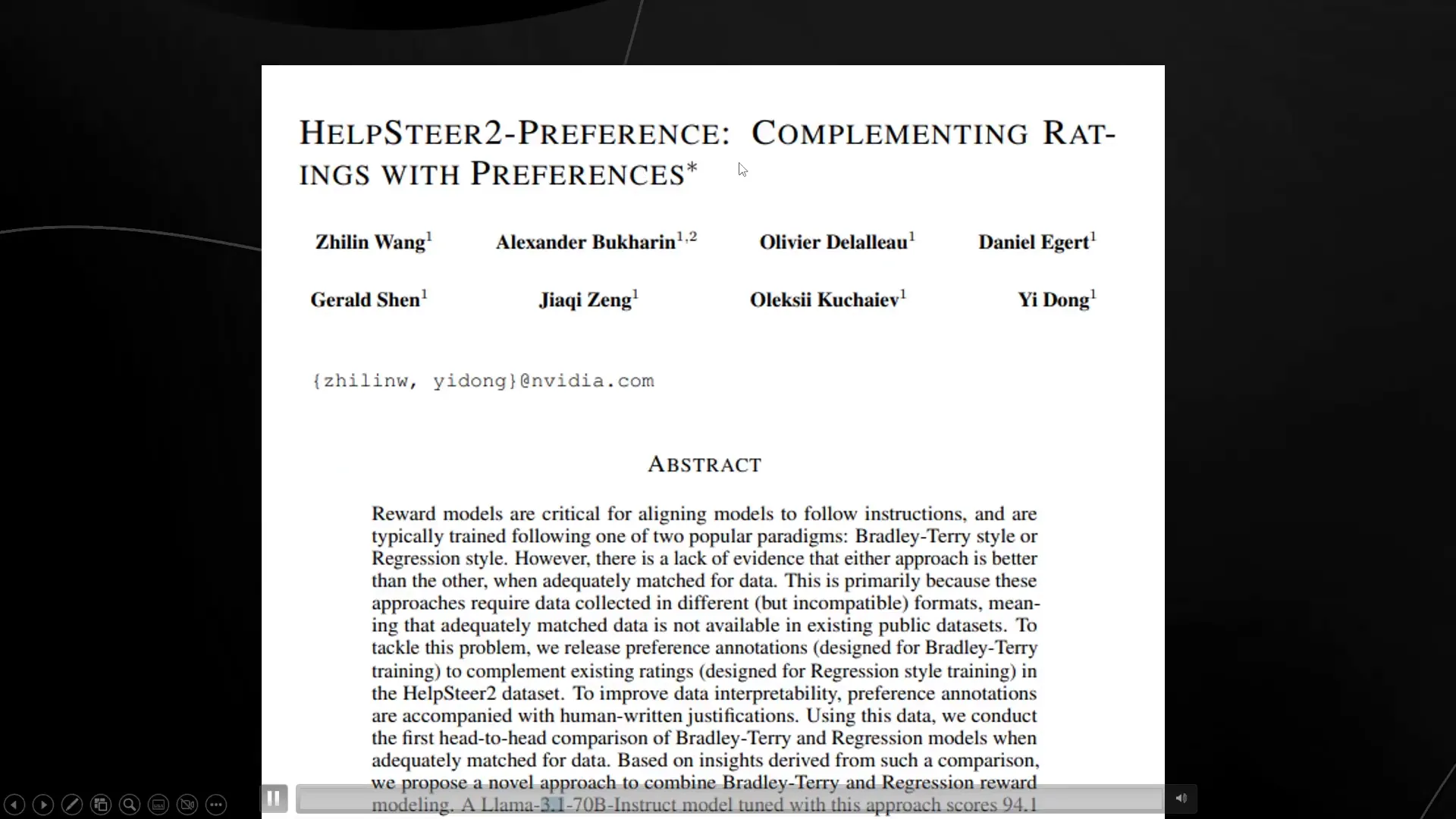
To close the gap, NVIDIA utilized a novel dataset named Help Steer 2 which comprises not only preference rankings but also numerical ratings for each pose. This advanced dataset supplies a more complete comparison, thus the combined reward model gains top scores on the Reward Bench benchmark. The deployment of the combined reward is the principal cause of Llama 3.1 Neaton outcompeting current cutting-edge systems.
Performance Evaluation Using Arena Hard Auto
The NVIDIA models were also included in the Arena Hard Auto, a tool for testing language models (LLMs). Automated tests based on instructions are the model and tool that open AI uses to check the performance of LLMs. The model performed well for the Chatbot Arena competition, with the only other model, GPT-4 Turbo, serving as the benchmark for comparison.
It is really interesting to see such results. The Llama 3.1 Neaton turbo engine obtained a slight 4% victory over GPT-4 Turbo, which is a strong indication of its successful performance in the area of AI. Nevertheless, it should be kept in mind that the result will essentially be different if style control is present in prompts, which actually changes how users judge the response.
Real-World Testing and Reasoning Challenges
While the benchmark is vital, how the model copes with practical questions is another significant thing to bear in mind. The Llama 3.1 Neaton, in a test that contains reasoning-based questions, was for a moment confused by such a question that mentioned irrelevant details. This issue can be seen in many AI models, such as OpenAI’s flagship model, GPT-4.
Just for the reason to enhance the model’s deliberation skills, really easy although a very effective method was used: model is asked to \”reread the question\” by the teacher. This small prompt had a marked impact and displayed perfectly the necessity for the careful provision of the question to obtain the fidelity of a correct response by the model.
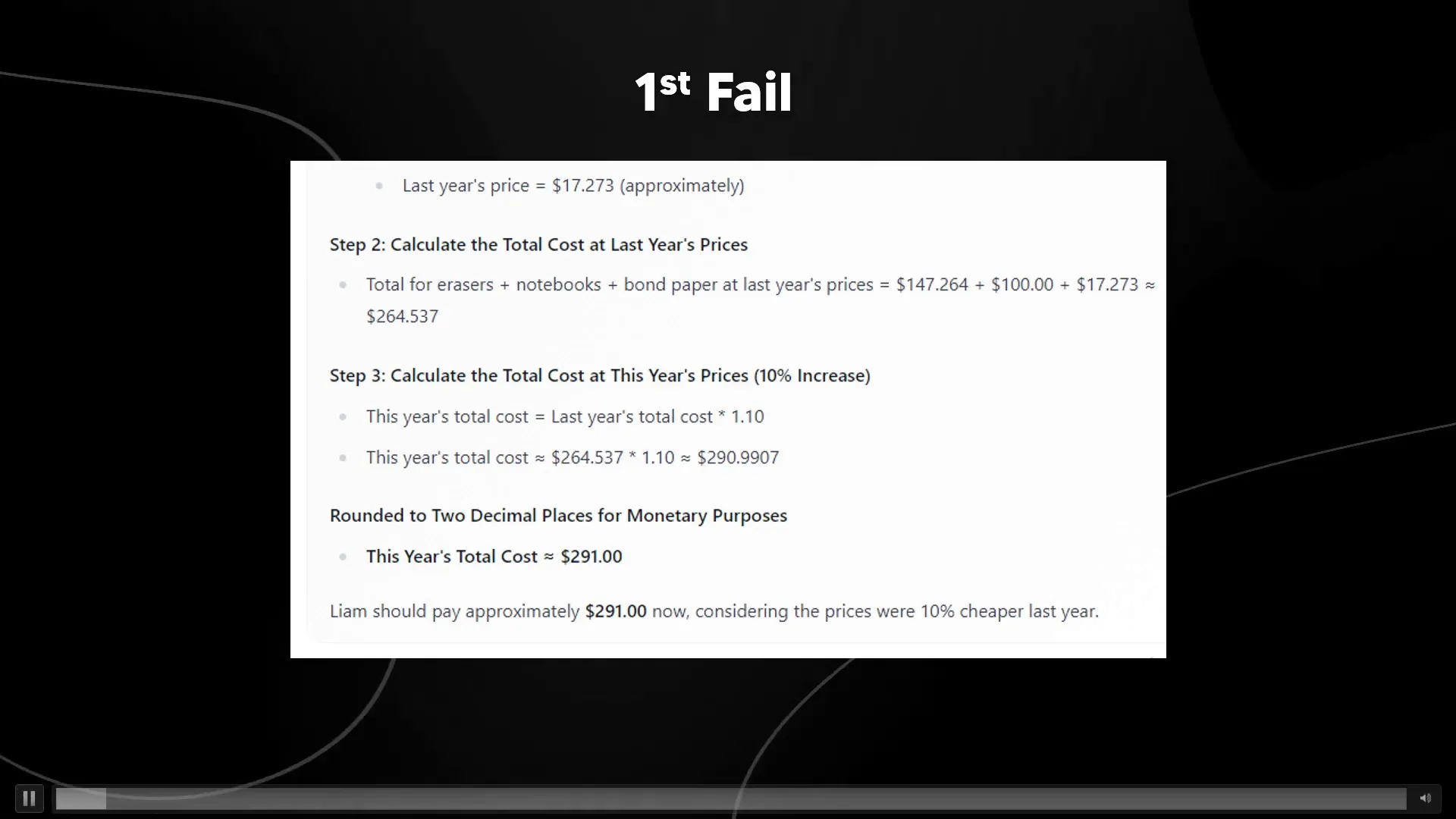
Impressive Reasoning Abilities
The Llama 3.1 Neaton was able to show its reasoning abilities even in more detailed tests by displaying correct counting and a general understanding of the situation. For example, the machine accurately gave the total number of kiwis, even though each was of a different size, which means it can think more independently now.
This ability to reason (ratio = 0) {?when speaking of?} can be attributed to the advanced reward modeling techniques employed during training. By prioritizing clear and logical responses, NVIDIA has positioned the Llama 3.1 Neaton as a formidable contender in the AI space.
The Future of Open Source AI
The report on Llama 3.1 Neaton’s win shows a promising trend for the future of open-source AI. With the advent of larger frontier models, we are likely to get a significant leap in terms of both reasoning and efficiency beyond kat’s capabilities. The fact is that projects based on open-source models are not limited to those that use proprietary software but to the ones that break the limits of AI Artificial Intelligence.
In conclusion, the Llama 3.1 Neaton 70B instruct model is a significant step forward for open-source AI technology. The NVIDIA company has achieved training methods, reward modeling, and performance that are unexpected. They are so awesome that they set the new standard among other companies which needs to follow them.
You’ve been asked to write a memory subtest for the Llama 3.1 Neaton model. Have you been able to test-write the Llama 3.1 Neaton model? Leave your thoughts and experiences in the comments below!